Innovation at ACC | Automated Data Abstraction Utilizing Artificial Intelligence and Natural Language Processing
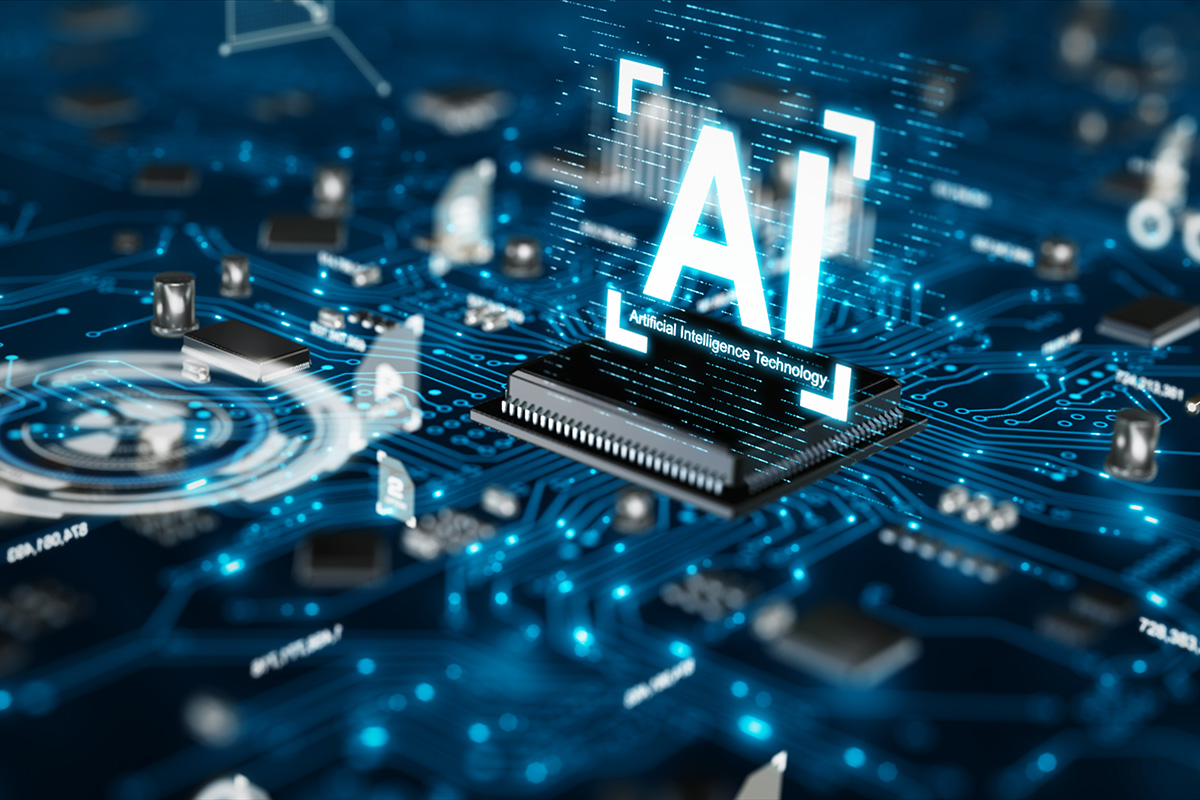
Promising solutions are available for efficient and accurate clinical data abstraction. Organizing information and bringing insights forward is a strength of software in the age of machine learning (ML) and artificial intelligence (AI).
Human and machine collaboration has the potential to maximize the potential of registries while liberating personnel from harmful tedium and freeing them to refocus on more engaging and valuable work.
Observational registries have been used for decades to define care standards based on the systematic aggregation of data with relatively low cost and less time than randomized trials. Although registries have implemented nationally accepted data standards, this is not to a level that supports semantic interoperability between registries and their source data systems.
Linking registries or accessing external data such as publicly available reference data sets from federal government agencies is also uncommon. There is an increasing demand for the development of cross-cutting performance measures that require data spanning multiple registries.
Reports are emerging that the quality of data is limited and describe stalled returns on investment for clinical registries to improve care. However, industries and regulatory bodies will continue to rely on registries for policies involving remuneration, ratings, key performance indicators, and best care practices.
Manual Data Abstraction is the Rate-Limiting Constraint
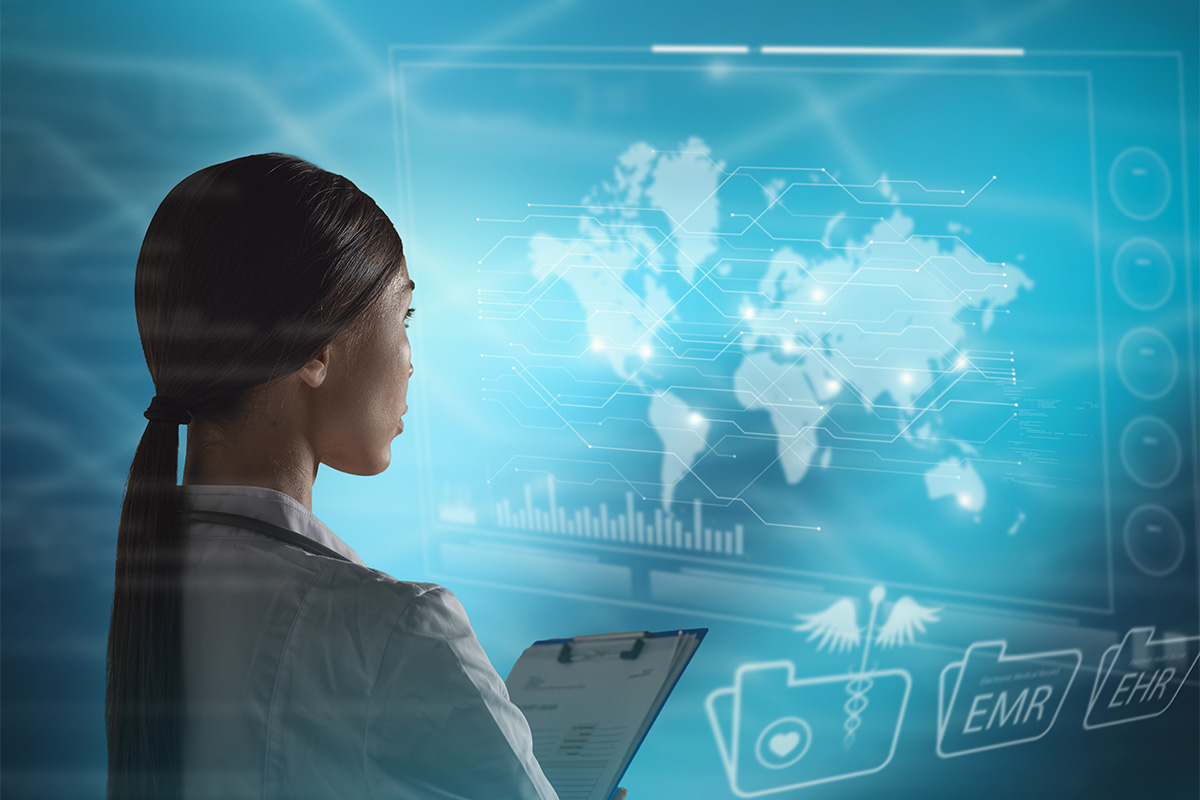
The quality of the solutions we come up with will be directly proportional to the quality of the description of the problem we are trying to solve. Herein lies the registry conundrum problem: limitations in how we collect data for registries importantly translate to constraints in what we can learn from registries.
Most observational registries operate in a mixed data collection environment due to the lack of structure and standardization of electronic health record (EHR) data. Continued dependence on data entry through manual chart abstraction also persists as standard practice, despite its shortcomings.
The efforts of the hospital personnel responsible for data abstraction from the EHR are often a key driver in the decisions on what data to include in a registry. This usually limits data capture to only consensus-derived EHR variables, which typically characterizes a small fraction of the patient information within the EHR.
The instance of manual data abstraction for registries may be a problem well-suited for artificial intelligence (AI). Natural Language Processing (NLP), a subset of AI, is the overarching term used to describe the process of using computer algorithms to identify key elements in everyday language and extract meaning from unstructured spoken or written input and is an essential tool for modern-day organizations. Modern industries use NLP routinely. It covers a range of needs, from data acquisition and processing to analytics, entity extraction and fact extraction.
With observational registries, where the collected patient variables are prespecified and rigorously defined, the application of NLP to automate chart abstraction is both pertinent and novel.
The source of truth recounting the narrative of a patient's hospital journey – from diagnosis, procedures, therapeutics, complications and resource utilization – are captured through a combination of values (structured data) and free text (unstructured data).
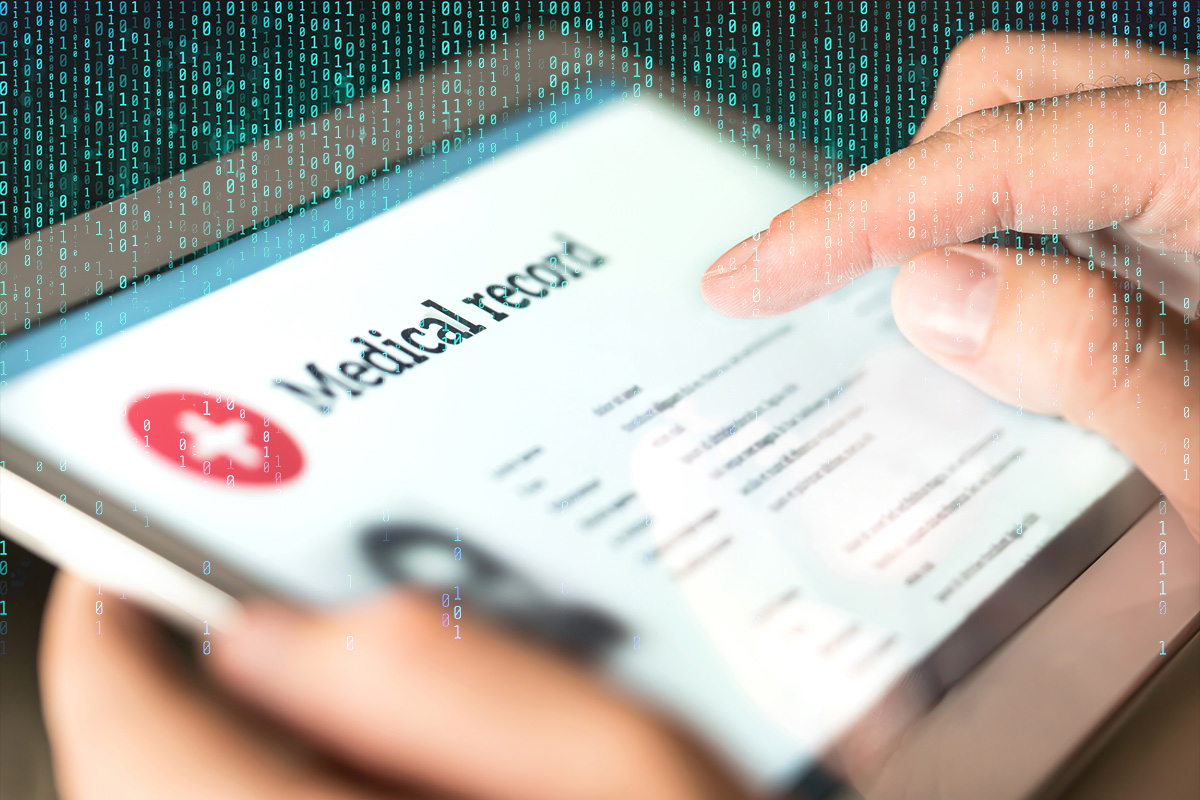
Curating the data from the EHR may be mitigated through the careful employment of NLP. When partnered with a human abstractor to make the final adjudication, limitations in data collection created by pure manual abstraction no longer apply while at the same time hospitals maintain control of the abstracted data.
Registries can rewrite their data dictionaries where the amount of structured and unstructured data captured is a matter of engineering rather than hiring more expensive data abstractors.
The technology may enable an essential pivot in the quality and depth of patient capture from an a priori hypothesized selection of relevant variables to data universes that are significantly more expansive in performing a posteriori research.
The technology also affords more contemporary interoperability standards integrating multiple disparate, but related registries that span the spectrum of disease across several hospital encounters, promoting the concept of centralization and vertical integration of information.
Finally, a more comprehensive integrative data universe may bring within reach a more suitable technological platform for comparative effectiveness research and randomized trials within a registry, in concert with unique research methodologies such as machine learning, neural network and deep learning.

Summary
Contemporary tools such as NLP/AI can improve data abstraction's efficiency and effectiveness across registries through automation. NLP/AI can enhance the value proposition for hospitals to participate in current and upcoming registries.
This approach can lessen the burden and cost of manual data abstraction, improve access for hospitals to their data and unlock the true potential of EHRs for quality improvement and clinical research.
Clinical Topics: Cardiovascular Care Team
Keywords: Natural Language Processing, Electronic Health Records, Artificial Intelligence, Comparative Effectiveness Research, Quality Improvement, Standard of Care, Consensus, Remuneration, Information Systems, Data Collection, Abstracting and Indexing, Innovation
< Back to Listings